AI-triaged 3D Pathology
Innovations in medical imaging techniques more and more medical information to be gathered from a single biopsy sample, but pathologist review of such large datasets becomes prohibitively time intensive. This technology provides an AI-driven method for the triage of a 3D pathology dataset for review by a doctor, with areas of concern elevated to the doctor’s attention for review in order to detect disease faster and more accurately.
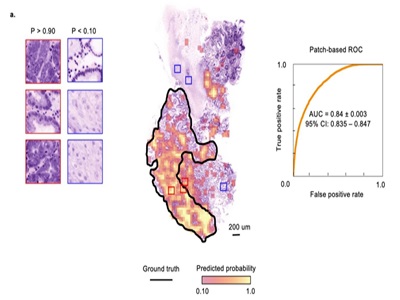
What is the Problem?
As the information available to pathologists from biopsy specimens increases, the importance of identifying the most important data for pathologist review becomes paramount for timely analysis of each sample. In an example case, prevention of esophageal cancer requires the review of endoscopic screening biopsies for tumors. Through the use of open-top light-sheet microscopy, a 3D visualization of the biopsy specimen can be non-destructively generated from a sample. This greatly improves the amount of information available as compared to conventional slide-based histology, typically consisting of 4 to 16 2D images (~1% of the total sample). Slide-based histology also results in the destruction of the sample, precluding any additional analysis of areas not selected for review. . This increase in information should increase the likelihood that cancer can be detected. However, there is an unmet need in the processing of this type of 3D dataset to identify the most important information for pathologists to review, as the human analysis of the entire 3D dataset for every biopsy is impractical.
What is the Solution?
The technology is a two-step data driven method for the triage of a 3D pathology dataset into a manageable number of 2D image sections as would be given in conventional histology, while maintaining the advantages of increased data available from modern methods such as the OTLS platform.
The first step is the creation of a 3D heatmap of the biopsy sample, indicating the likelihood of disease at every point across the sample. This could be done through the aggregation of predictions generated by a deep learning model trained on pathologist annotations. The second step is the identification of 2-dimensional sections with the greatest probability of containing disease tissue, using the 3D heatmap as data for a method such as a classification algorithm. The final output is a set of 2D medical images for pathologist review with the highest likelihood of containing the tumor or other disease markers, reducing pathologist workload while increasing the diagnostic sensitivity.
What is the Competitive Advantage?
This technology is built on the OTLS platform for analysis of biopsy samples, making the input data for the technology higher quality than the data collected via conventional slide histology. This allows for non-destructive collection of entire 3D pathology datasets, as opposed to limited cross-sectional images from slide mounted samples.
The two-step method for analyzing the resulting information-dense 3D dataset both increases the diagnostic sensitivity of screenings such as the crucial endoscopic screening for EAC prevention and reduces the workload on pathologists to the analysis of only the most important 2D sections.
This triage of 3D data into 2D sections also outputs data in a format familiar to pathologists: a set of 2D images that can be false-colored to appear like conventional slide-histography samples. This minimizes the retraining of pathologists needed for clinical adoption of the method and retains a paradigm that keeps the pathologist "in the loop".
-
swap_vertical_circlemode_editAuthors (1)Jonathan Teng-Chieh Liu
-
swap_vertical_circlelibrary_booksReferences (1)
- Lindsey A. Erion Barner, Gan Gao, Deepti M. Reddi, Lydia Lan, Wynn Burke, Faisal Mahmood, William M. Grady, Jonathan T.C. Liu (2023-12), Artificial Intelligence–Triaged 3-Dimensional Pathology to Improve Detection of Esophageal Neoplasia While Reducing Pathologist Workloads, Modern Pathology, 36, 100322
-
swap_vertical_circlecloud_downloadSupporting documents (1)Product brochureAI-triaged 3D Pathology.pdf